In the fast-paced world of AI, leaders constantly face the challenge of deciding whether to build AI solutions in-house or to purchase them from vendors. During our panel discussion at AI Dev Summit 2024 in San Francisco we gathered AI leaders from PayPal, Chime, and Gusto to uncover the decisions these leaders go through when deciding on building vs buying AI solutions.
This is the third article in our three-part series, in which we uncover the insights of Kartik Khana, Head of Data Science, Machine Learning, and AI at Gusto. During the panel session, Kartik shared his experience transitioning from traditional machine learning to a complete focus on generative AI. The panel was moderated by Remy Thellier, Head of Partnerships at Vectice.
You can read the first articles with Khatereh Khodavirdi, Vice President of Consumer Global Growth Enterprise for Data Science at PayPal on Navigating the AI Build vs. Buy Decision and Philip Denkabe, Head of Data Science at Chime, who shared his approach to prioritizing speed and efficiency in AI development.
About Kartik Khanna

Kartik is a seasoned expert in applied NLP with over 15 years of experience in healthcare, property casualty insurance, and fintech. He has built and led ML teams focused on risk, fraud detection, and data extraction. Currently, he leads Gusto’s Generative AI strategy and initiatives across the enterprise, emphasizing customer-facing applications and operational efficiency.
Kartik Khanna will share his invaluable insights on the strategic considerations of buying versus building evaluation platforms in the rapidly evolving landscape of Generative AI.
Remy Thellier’s key Takeaway
“From my conversation with Philip, I gained several key insights for teams growing fast:From my conversation with Kartik, I gained several key insights, specifically about keeping pace with the evolving generative AI landscape.
- Large language models are now a commodity, with streamlined APIs making iteration easier. The key differentiator is having a robust evaluation platform to ensure performance, reliability, and accuracy. This enables continuous improvement and alignment with business goals.
- Continuous evaluation of whether to build or buy AI solutions is crucial for agility and staying updated. Careful evaluation of new tools and vendors is vital, focusing on the team's specific needs and how new solutions fit into existing infrastructures. This helps distinguish effective tools from less useful ones.
- Managing customer-facing AI applications requires clear metrics and continuous monitoring to ensure a seamless user experience and manage risks like hallucinations and latency."
Kartik’s Top 6 Tips for Leaders Making Build vs. Buy Decisions
1. Keeping Up with Rapid Innovation in the Generative AI Space
Kartik highlights the rapid pace of innovation in the AI field, especially in generative AI. New tools and functionalities emerge frequently, making it essential to stay updated. He emphasizes the need to continuously evaluate whether to build or buy solutions, considering the evolving landscape.
"In the world of AI, the pace of innovation is remarkable. New tools and functionalities are being introduced every month, continually changing the landscape.”
Kartik mentioned that this rapid pace means that teams must be agile and willing to adapt quickly. He added: "Building is not just going to be, I build it once, and then it runs on autopilot for the remaining 12 months; somebody has to own it, maintain it, and keep updating it."
2. Encouraging Intentional Learning
Q: How do you ensure your team keeps up with new developments without getting distracted?
To keep up with the latest developments, Kartik's team carves out intentional time for learning and exploration. This approach ensures that team members remain curious and aware of new technologies without getting tunnel-visioned by their current projects.
"We try to carve out intentional time. So people can go out and take courses and be more curious about what else is out there," he explained.
Additionally, Kartik mentioned that companies often reach out with new tools and technologies, making it essential to stay open to evaluating new options while focusing on immediate needs.
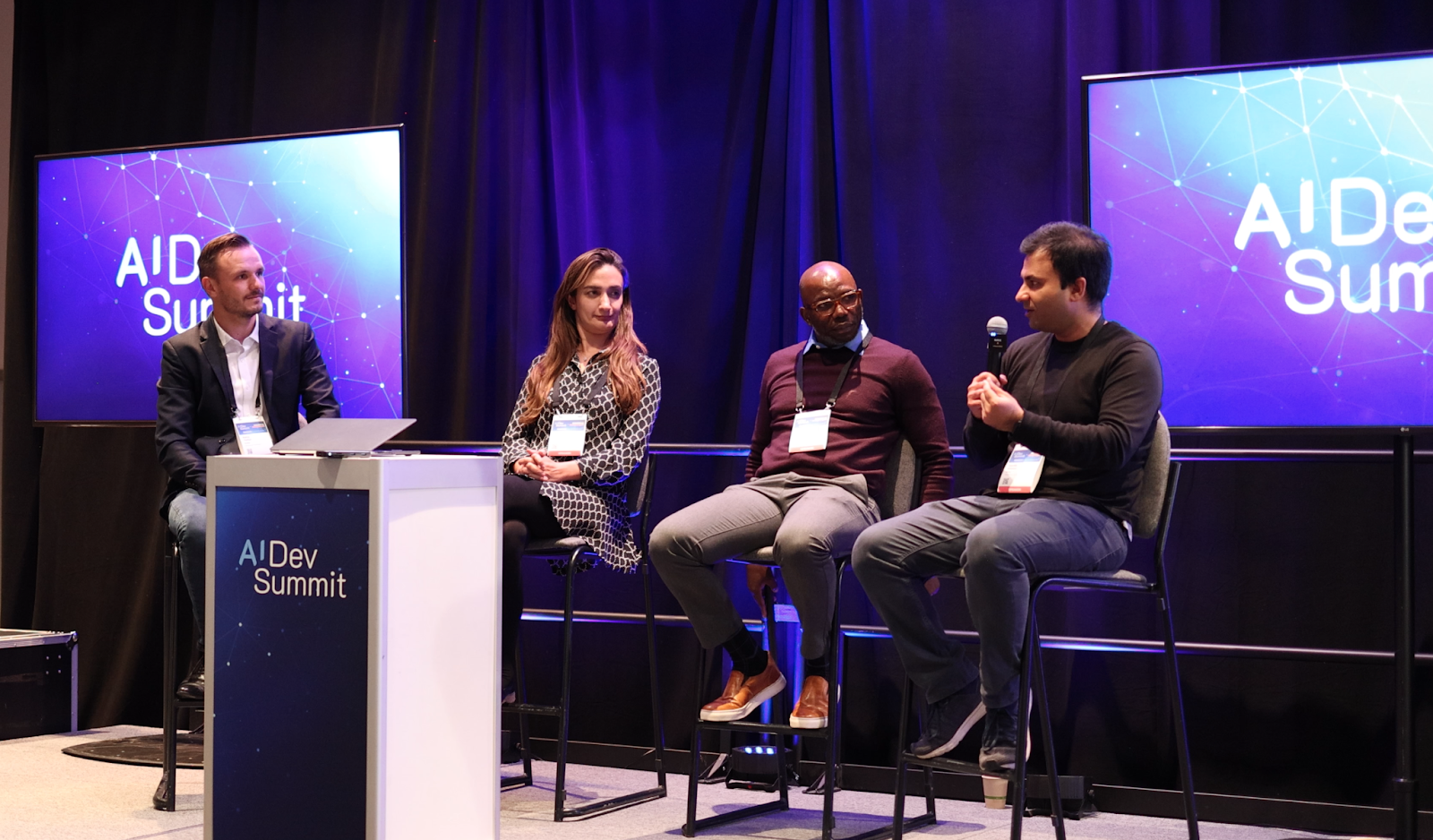
3. Evaluating New Tools and Vendors
Q: What makes evaluating tools and vendors particularly challenging in the generative AI space?
With the increasing number of vendors offering generative AI solutions, Kartik stresses the importance of careful evaluation. Distinguishing between effective tools and shiny new toys requires a clear understanding of the team's needs and the potential impact on their workflow.
"The rapid pace of change means that many vendors are constantly introducing impressive new tools. How do you stay informed about what's truly effective?" he said.
Kartik also emphasized the importance of being clear about assumptions and understanding how new tools fit into existing infrastructures to avoid unforeseen complications.
4. Building Customer-Facing AI Applications
Q: How do you handle the unique challenges of using generative AI for customer-facing applications?
Gusto uses generative AI for both business efficiency and customer-facing applications. Kartik explains the unique challenges of ensuring a seamless user experience while managing risks like hallucinations and latency.
"How do we assess value? You can easily spend so much time iterating on a solution, building something, but then you ship it, people start using it, like having a very clear sense of what metric are you solving for. And then how would you measure success?" He elaborated: "In the spirit of moving fast, we don't want to break things."
He emphasized the need for robust evaluation metrics and continuous monitoring to ensure the AI solutions meet user expectations and business goals.
5. Balancing Use of Open Source vs. Building Proprietary Models
Q: How do you balance the use of open-source models with proprietary solutions?
Kartik discusses the benefits of using open-source models for quick deployment and iterative development. However, he also points out the importance of thorough evaluation on their own data to ensure these models meet specific needs.
"Open AI out of the box does amazingly well, for a general use case. Now over time, you might find that with enough data, you may want to fine-tune your application," he noted.
This approach allows teams to quickly start with open-source solutions and gradually transition to more customized models as they gather more data and insights.
6. Implementing Continuous Evaluation
Q: What strategies do you use for continuous evaluation of AI models and tools?
As models and tools evolve, continuous evaluation becomes a critical practice. Kartik's team focuses on both offline and production evaluations to ensure their solutions perform as expected and adapt to real-world usage.
"The more you can de-risk upfront, the easier your life will be in production," Kartik emphasized.
He mentions the importance of having systems in place for real-time monitoring and feedback to identify and address any issues that arise in production quickly. "Having the right systems in place so you can react fast becomes the top-of-mind thing."
Final Thoughts
Kartik's insights provide a roadmap for teams navigating the dynamic generative AI landscape. By staying updated, dedicating time for learning, carefully evaluating tools, managing customer-facing applications, leveraging open-source models, and prioritizing continuous evaluation, AI leaders can adapt and thrive.
His emphasis on curiosity and clear metrics underscores the need for a proactive and strategic approach in this rapidly changing field.
Get all the insights
Get to know the speakers
By Alexander Gorgin, Analyst at Vectice